In the rapidly evolving digital marketplace, businesses constantly seek innovative ways to enhance customer experience, streamline operations, and, most importantly, boost sales. One of the most transformative advancements in recent years has been the integration of artificial intelligence (AI) in eCommerce. Specifically, AI-driven product recommendations have emerged as a powerful tool to personalize the shopping experience and drive revenue growth.
This article explores how AI-powered product recommendation engines work, the benefits they offer, and how businesses can leverage them to increase sales. Let’s dive into the mechanics, best practices, and potential future of AI in product recommendations.
1. What Are AI-Driven Product Recommendations?
AI-driven product recommendations are suggestions provided to users based on data-driven insights and algorithms that analyze consumer behavior, preferences, and purchase history.
Powered by machine learning (ML) and natural language processing (NLP), these systems predict what products or services a customer is likely to be interested in, creating a personalized shopping experience.
There are multiple types of recommendation systems, including:
- Collaborative filtering: This method recommends products based on the behavior of similar users. If user A and user B have both purchased Product X, and user A also buys Product Y, the system may suggest Product Y to user B.
- Content-based filtering: Here, the system suggests products based on the attributes of items a user has already purchased or shown interest in.
- Hybrid models: Many modern eCommerce platforms use a combination of both methods for more accurate and personalized recommendations.
2. The Power of Personalization in eCommerce
Personalization in eCommerce is more than just addressing customers by their first name. It’s about understanding their needs, preferences, and behaviors to offer relevant product suggestions that enhance the shopping experience. AI-driven recommendations are at the heart of this personalization effort.
Why Personalization Matters
- Increases Customer Engagement: Shoppers are more likely to interact with products that are relevant to their preferences. A personalized recommendation helps engage users, reducing bounce rates and increasing the time spent on a website.
- Improves Conversion Rates: By showing customers items they are likely to be interested in, AI recommendations can improve the likelihood of conversion. A study by Barilliance found that personalized product recommendations account for up to 31% of eCommerce site revenues.
- Fosters Customer Loyalty: When customers feel that a brand understands their preferences, they’re more likely to return. This sense of being catered to fosters brand loyalty, increasing repeat purchases and lifetime customer value.
- Enhances Cross-Selling and Upselling: AI systems are effective in suggesting complementary products (cross-selling) or higher-value alternatives (upselling), which leads to increased average order values.
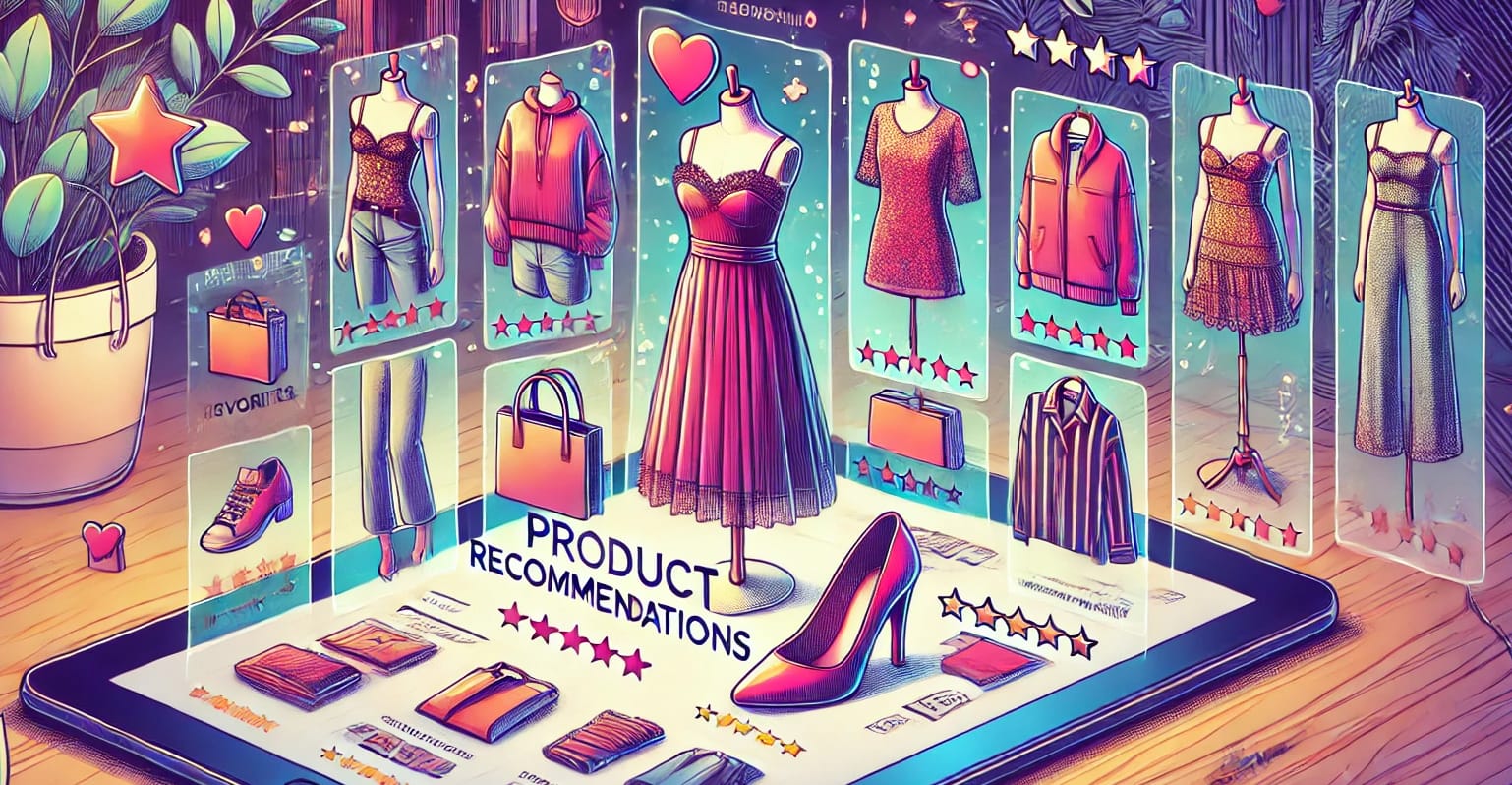
3. How AI-Driven Recommendations Work
AI-driven recommendation systems leverage large volumes of data to learn and make suggestions. Here’s a breakdown of how they typically work:
Data Collection
The first step is data collection. AI systems gather customer data from various sources, including:
- Browsing history
- Search queries
- Past purchases
- Social media interactions
- Product reviews and ratings
- Demographic information
Data Processing and Analysis
Once data is collected, machine learning algorithms analyze it to identify patterns, trends, and correlations. The AI system can process complex relationships between different data points, enabling it to predict customer preferences with remarkable accuracy.
Personalized Recommendations
After processing the data, the system generates personalized recommendations for each user. For example, if a customer has recently viewed several hiking boots, the AI might suggest outdoor gear such as backpacks or weather-appropriate jackets. These recommendations can appear in various forms:
- Product suggestions on the homepage or product page
- Recommendations via email or SMS
- Cross-sell and upsell suggestions in the shopping cart
- Reminders of previously viewed or saved items
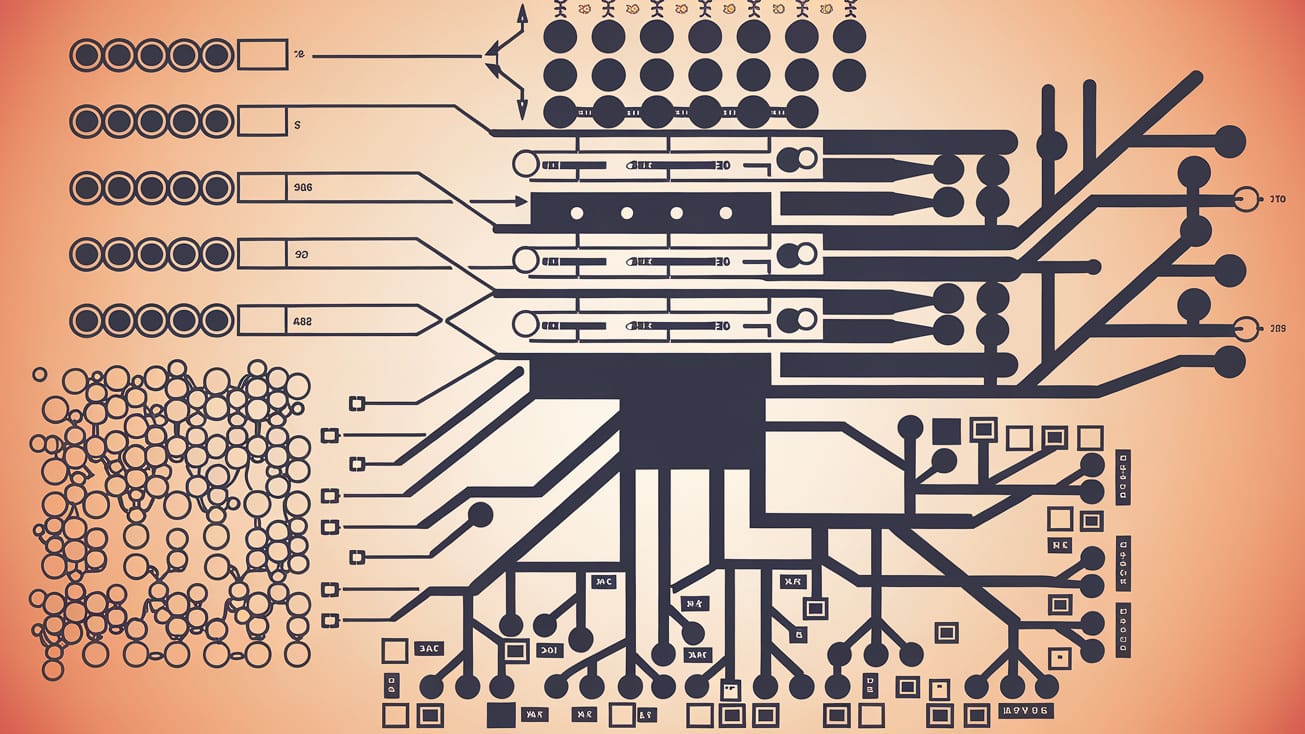
4. Benefits of AI-Driven Product Recommendations for Businesses
The advantages of incorporating AI-driven recommendations into an eCommerce strategy are numerous. Let’s explore the key benefits for businesses:
- Increased Revenue
AI-driven product recommendations directly impact a company’s bottom line. According to McKinsey, 35% of Amazon’s revenue comes from product recommendations. By showing customers products they are more likely to purchase, businesses can increase both conversion rates and average order value.
- Improved Customer Experience
A positive customer experience is critical to business success. AI-powered recommendation engines provide seamless, personalized shopping journeys that make customers feel understood and valued. This enhances their overall experience and increases satisfaction.
- Enhanced Decision-Making
AI systems continuously learn from customer data, which means the more customers engage with the platform, the more refined the recommendations become. This allows businesses to adapt their strategies in real time, ensuring that product offerings remain relevant.
- Scalability
Unlike manual recommendations, AI-driven systems can handle vast amounts of data and customers simultaneously. This scalability allows businesses to offer personalized experiences to millions of users without additional human resources.
- Reduced Cart Abandonment
One of the major challenges in eCommerce is cart abandonment. AI systems can counter this by providing timely and relevant product suggestions, encouraging users to complete their purchases. Additionally, AI can send automated reminders or follow-up emails with recommendations based on the items left in the cart.
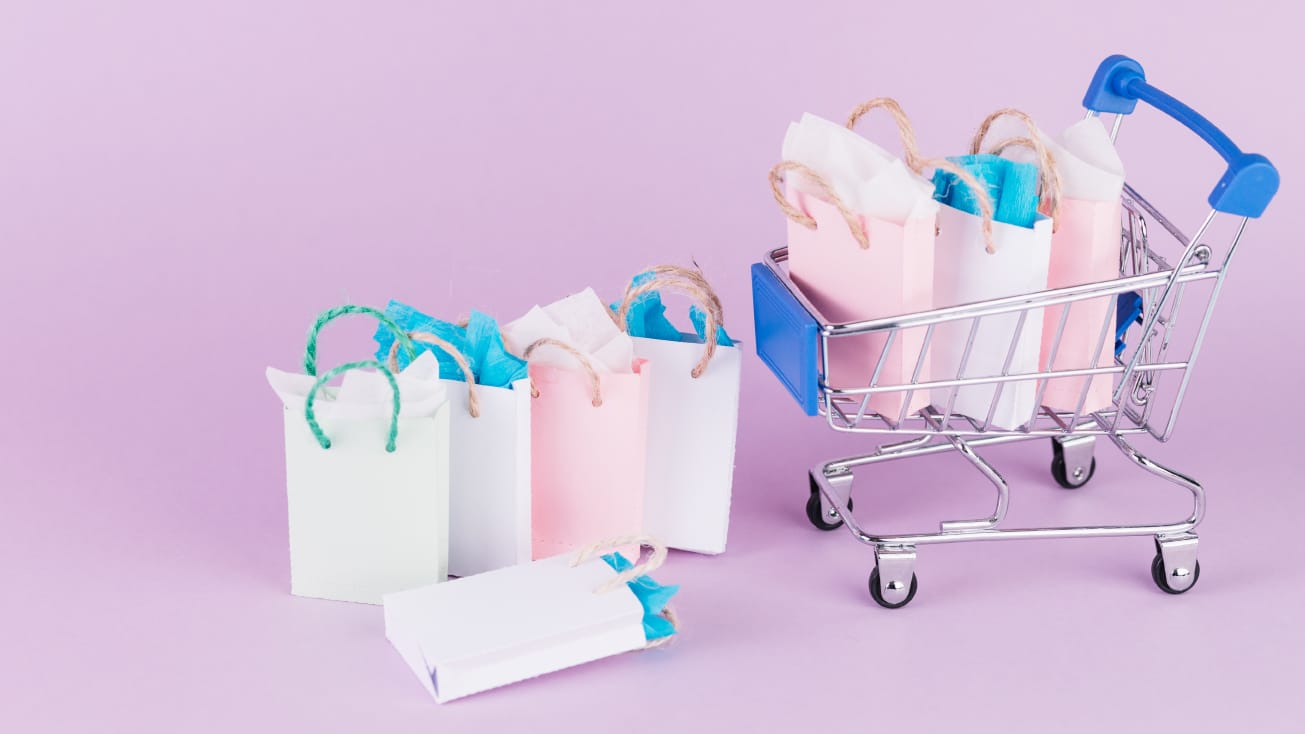
5. Best Practices for Implementing AI-Driven Product Recommendations
To maximize the impact of AI-driven recommendations, businesses must follow best practices in their implementation. Here are a few key strategies:
1. Ensure Data Quality
The effectiveness of AI recommendations depends on the quality of the data fed into the system. Incomplete, outdated, or inaccurate data can lead to poor recommendations, which may frustrate users. Businesses should prioritize collecting comprehensive and up-to-date data on customer behavior, preferences, and demographics.
2. Balance Personalization with Privacy
While personalization is key to driving engagement, it’s essential to respect user privacy. Transparency about data collection practices and providing users with the ability to opt out of data sharing is critical. Failing to protect user data can damage a brand’s reputation and lead to legal repercussions.
3. Test and Optimize
AI systems require continuous testing and optimization to ensure their effectiveness. A/B testing can be used to assess the impact of different recommendation strategies on conversion rates and customer satisfaction. AI-driven tools can then be adjusted based on these results for better performance.
4. Leverage Different Recommendation Types
In addition to providing recommendations based on browsing or purchase history, businesses can explore different types of recommendations, such as:
- Social proof-based recommendations: “People who bought this also bought…”
- Personalized upselling: “You may like this premium version…”
- New arrival suggestions: “Check out the latest in this category…” Offering a variety of recommendations keeps the shopping experience dynamic and engaging.
5. Use Recommendations Across Channels
AI-driven recommendations should not be confined to a website or app. Brands should integrate them across multiple channels, including:
- Email marketing: Personalized product recommendations based on recent activity or past purchases.
- Mobile push notifications: Reminders or recommendations sent directly to the user’s mobile device.
- In-store assistance: AI can also power in-store kiosks or sales assistants to provide personalized recommendations in physical retail environments.
6. Challenges and Limitations of AI-Driven Recommendations
Despite their advantages, AI-driven product recommendations are not without challenges. It’s important to recognize these potential hurdles and prepare for them.
1. Cold Start Problem
One of the most significant limitations of recommendation systems is the “cold start” problem. This occurs when there is insufficient data on new users or products, making it challenging for the AI to generate accurate recommendations. Businesses can mitigate this by using hybrid models or incorporating demographic data and surveys to fill in the gaps.
2. Over-Personalization
While personalization is valuable, there’s a risk of over-personalization. If the system focuses too narrowly on a customer’s past preferences, it may miss the opportunity to introduce new or complementary products. A balance should be struck between offering familiar items and encouraging exploration.
3. Data Privacy Concerns
With increasing scrutiny on data privacy, businesses must handle customer data responsibly. Failing to comply with data protection regulations, such as GDPR or CCPA, can lead to fines and a loss of customer trust. AI systems must be designed with privacy in mind, using anonymization techniques and ensuring data security.
7. Future Trends in AI-Driven Recommendations
As AI technology continues to evolve, so too will its capabilities in product recommendations. Here are some trends to watch:
1. Voice and Visual Search Integration
With the rise of voice assistants like Alexa and Siri, as well as visual search technologies, product recommendations will likely evolve to accommodate these new search methods. Customers will be able to receive personalized recommendations by simply speaking or uploading images.
2. Real-Time Personalization
AI systems are moving towards real-time personalization, where recommendations are adjusted in real-time as customers interact with the site. This creates a more dynamic and engaging shopping experience, catering to customers’ needs and moods in the moment.
3. Predictive Analytics
AI will increasingly leverage predictive analytics to not only recommend products but anticipate future needs. For example, if a customer frequently purchases fitness equipment, the system may suggest related products even before the customer actively looks for them.
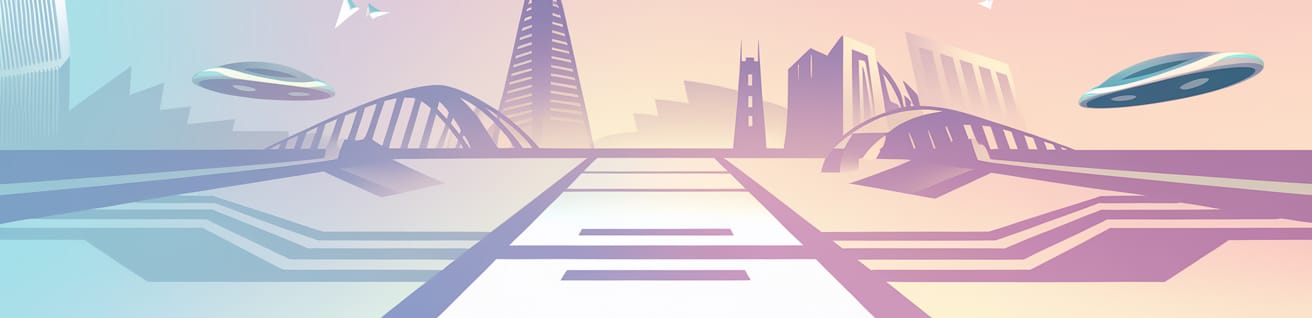
Conclusion: AI Recommendations – The Future of eCommerce Sales Growth
AI-driven product recommendations are a game-changer in eCommerce. By providing personalized, relevant product suggestions, they significantly enhance the shopping experience, increase customer loyalty, and ultimately drive higher sales. As AI technology continues to advance, businesses that embrace and optimize AI-powered recommendation systems will be well-positioned to lead in the competitive online retail space.
For companies looking to boost their sales, investing in AI-driven recommendations is not just a trend – it’s a necessity. Whether you’re a small business owner or a global eCommerce giant, AI holds the key to unlocking unprecedented growth in the digital age.